From code to clinic: The challenges in translating machine-learning models into real-world products
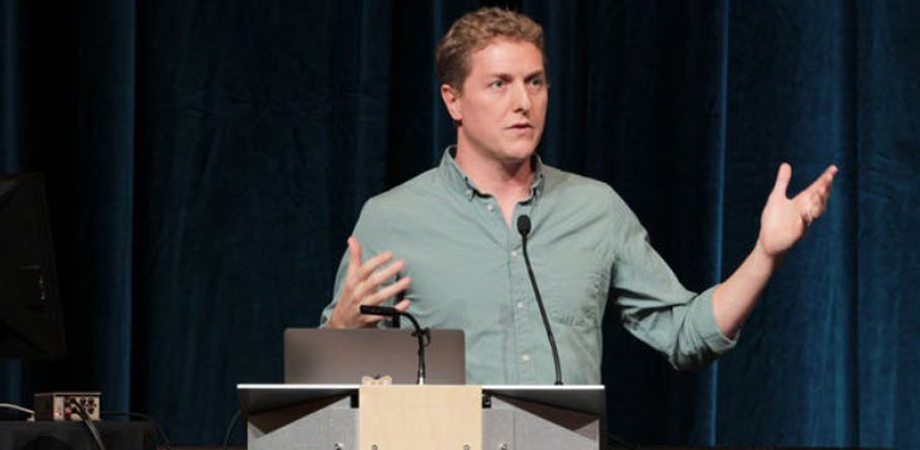
Inspired by the potential of artificial intelligence (AI) to improve access to expert-level medical image interpretation, several organizations began developing deep learning-based AI systems around 2015. Today, these AI-based tools are being deployed at scale in various areas around the world, often bringing screening to populations lacking easy access to timely diagnosis. The path to translating AI research into a useful clinical tool has gone through several unforeseen challenges along the way.
At SPIE Medical Imaging 2023, Dale Webster, a research director at Google Health, will share some lessons contrasting a priori expectations (“myths”) with synthesized learnings of what truly transpired (“reality”), to help others who wish to develop and deploy medical AI tools.
What are some of your responsibilities as a research director at Google Health?
My team at Google works in areas where AI can help doctors and caregivers provide better care for their patients. We share our learnings in the form of papers, studies, and small-scale deployments so that others can build on our work. Much of my time is spent partnering with leaders in the healthcare space to explore these applications in a safe, equitable, privacy-preserving manner.
You were a software engineer previously. Does that give you a different perspective than colleagues with a medical background?
I find that sometimes I default into a “technology-first” approach — I get excited about the latest and greatest AI technology and start looking for a problem I can apply it to. I learned the hard way that if I want to solve the important problems in this space and help those who need it most, I need to partner closely with folks who understand healthcare deeply. That is now a basic tenant of how I work.
Your abstract notes that “The path to translating AI research into a useful clinical tool has gone through several unforeseen challenges along the way.” What are some of these unforeseen challenges? How are they being met?
The challenges I’ll be talking about are very practical ones — lessons learned the hard way about the importance of having a deep understanding of the problem you’re trying to solve, and the value of digging in to understand the underlying data that you’re working with. In general, we’ve met these challenges through an iterative process of failing in some embarrassing way, then learning from our mistakes and moving forward a little wiser than we were before.
What do you see as the future for artificial intelligence in medical imaging? What would you like to see?
I think the big challenge facing us right now is that we academic researchers have all of these models in hand that we’ve shown to be effective in controlled scenarios. How do we go from there to a future where every day, patients around the world are receiving the benefits of this technology? In particular, how do we make sure that the benefit goes to those who need it the most? I’d like to see us as a community make that last part a priority, and to have it become second nature when we are thinking about the impact of our work.
What would you like attendees to learn from your talk?
I believe that now is the time to use AI to build real tools that help real people with real problems. I hope that by sharing the difficult lessons we’ve learned in our first attempts at achieving this goal, we can help enable many more successes in the coming years.
Enjoy this article? Get similar news in your inbox |
|