Let there be smart light
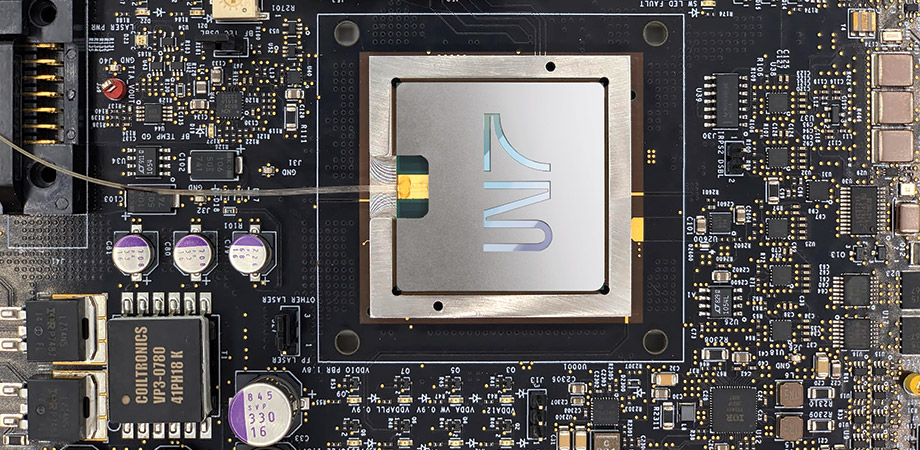
Ever since computers evolved beyond purely analog mechanical devices in the 1940s, they've operated by moving and manipulating electrons, whether in vacuum tubes, transistors, or silicon chips. The state of technology didn't allow any other reasonable possibilities, and there really wasn't any pressing need for some. But in a 21st-century world becoming ever more dependent on crunching ever more data at faster and faster rates to support artificial intelligence (AI) applications, a new approach is taking hold: Faster and more efficient computing using photons.
The basic idea of optical computing has been around for decades, but its new incarnation is more properly termed photonic computing. As Nicholas Harris, founder and CEO of Lightmatter, a photonics computing startup, puts it, "The word optical kind of harkens back to more of bulk optics, free space lenses and mirrors, that sort of thing. Photonics is more of an integrated platform: The word photonics is parallel to electronics."
Simply defined, optical/photonic computing uses photons to calculate a desired function or mathematical operation.
The early attempts at optical computing technology, in the late 1970s and early 1980s, turned out to be not quite ready for prime time, mostly because, as Andrew Rickman of Rockley Photonics remarked in a presentation remarked in 2018 at SPIE Photonics West, "Photons behave differently from electrons."
That fact posed serious problems when Bell Labs researchers experimented with creating an optical transistor. "In the '80s, Bell Laboratories was claiming that they had come up with an optical computing technology," Harris says. "They were working on an optical transistor. Transistors are nonlinear, which means that you can do things like create logic statements—if this/then, that sort of thing. It allows you to write programs using those statements. That's what digital computers are really good at. What they found is that you really couldn't string together very many logic operations before you lost all your signal, and that there were a bunch of challenges in trying to build logic with optics."
As Ryan Hamerly, a visiting scientist at MIT's Quantum Photonics Laboratory, wrote in an IEEE Spectrum article: "Nonlinearity is what lets transistors switch on and off, allowing them to be fashioned into logic gates. This switching is easy to accomplish with electronics, for which nonlinearities are a dime a dozen. But photons follow Maxwell's equations, which are annoyingly linear, meaning that the output of an optical device is typically proportional to its inputs." Aside from the significant loss of signal, there was also the problem of storing optical data.
At the time, the realities of the technology couldn't quite live up to the hype surrounding it, and it became clear that optical transistors weren't going to be replacing the traditional silicon variety anytime soon. Work using optical systems to explore quantum computing concepts continued in the 1990s with bulky free-space components such as lenses and lasers mounted on large breadboards, impractical for any kind of application.
"My background is in physics," Harris notes. "And I can tell you that you really don't want to do nonlinear operations using optics or photonics. Running operating systems and more general-purpose computing is not likely to be done fully using optics." Optical technology in the form of fiber optics may have revolutionized communications and the growth of the internet, but the computers and other devices it tied together still largely consisted of conventional microelectronics.
As the computing universe moves ever deeper into AI solutions for an increasing range of applications, however, photonic computing is presenting unique possibilities. It turns out that integrated photonics, in which photonic devices are incorporated on a microchip, is just the thing for the massively parallel processing needed for artificial intelligence systems (AI), machine learning (ML), and deep learning neural network calculations, offering far greater speed and computing efficiency while using far less power than electrons.
Lightmatter has developed a photonic chip called Envise, specifically designed for AI. "It isn't the type of computing that you would do to run an operating system or a video game," Harris explains. "It's for running neural networks. The computation that you're doing a lot of the time there is linear algebra, a lot of adds and multiplies. We're able to do those adds and multiplies using integrated photonic components." As a very broad mathematical tool used in modeling all sorts of real-world phenomena from rocket launches to financial transactions, linear algebra is also at the heart of deep learning algorithms. Those depend on a linear algebra operation called matrix multiplication, which lends itself quite well to the analog linearity of photonics. "AI is the first market we're going after because it's a very exciting market, and it has huge implications for humanity."
Renewed enthusiasm for dedicated AI processor chips was spurred in 2017 when Google announced its development of a deep learning processor called the tensor processing unit (TPU). Harris says, "It basically had an array of these matrix processors, 256 × 256 multiply-accumulate units. And with that, they were able to accelerate a lot of the core computations in deep learning. What we're doing is similar to Google's TPU work. We've replaced their electronic compute core with a photonic compute core. It saves a ton of power and allows you to run a lot faster."
Indeed, a major selling point for photonic computing is its greatly reduced power consumption. The proliferation of large data centers and cloud computing is demanding ever more of the world's energy while also contributing significantly to accelerating climate change. According to Harris, photonics can play a major role in changing that."So, let's say that AI gets to 10 percent of that. You're talking about two percent of the entire planet's energy consumption just on this specific type of program that you might run. What we're trying to do is reduce that environmental impact. Our technology allows you to run the same programs faster but using significantly less energy."
A Lightmatter photonic computing rack. Credit: Lightmatter
A key part of Lightmatter's Envise chip and other integrated photonics is a highly miniaturized version of a device called a Mach-Zehnder interferometer (MZI), which splits and recombines a collimated laser beam at the nanoscale. Large arrays of MZIs perform the matrix multiplication at the core of most deep-learning AI algorithms. "There's been a lot of innovation that's gone into the core compute element that goes into our processors," says Harris. The Envise chip, which Lightmatter claims is anywhere from five to 10 times faster than Nvidia's top-of-the-line A100 chip for AI, will be hitting the market this year, complete with a specially designed software stack that will allow plug-and-play compatibility with existing systems.
Lightmatter is only one of the players in what's becoming a rapidly expanding photonics computing market. Others include Luminous, Optalysis, and Lightintelligence, each pursuing their own variations on the photonic computing theme.
Some companies such as Ayar Labs in California are focusing not on the processor core at the heart of the computer but how that core moves data in and out. Instead of transforming the CPU of the computer to photonics, the idea is to speed up communications within the system and its connections with the outside world. "We use some of these same underlying technologies, but we're focused on solving different problems," says vice president and managing director Hugo Saleh. "There's a massive market opportunity here to open up these bottlenecks that have been preventing communication between chips. And this really ties into things like high-performance computing and AI."
Just as fiber optics enables faster communications than copper wires for internet and cable, it can do likewise inside a computer, where billions of components must connect and communicate as fast as possible. "There's something called SerDes [serializer/deserializer], which is the electrical signaling that goes from switch chip to a node," Saleh says. "That electrical signaling rate now is getting very difficult," he says, because it raises energy costs and introduces problems like heat that can mean difficult revisions to the engineering. "They went from 10 gig to 25, to 50 to 100, and they're jumping through hoops to try and figure out how to keep driving that signaling rate higher and higher. And there's a general consensus that the 100-gig generation is it."
Ayar's solution uses integrated optical input/output (I/O) chiplets that interface with larger chips to vastly increase data rates. Saleh says, "With an electrical wire, you can send one signal down at a time. With a fiber, you can send multiple frequencies down at the same time. In our case, we send eight colors of light all at the same time." That means eight times the amount of information can be sent down the same optical fiber.
Ayar Labs TeraPHYTM optical I/O chiplet. Credit: Ayar Labs
Just like the integrated photonics processor, optical I/O also offers a significant energy payoff. "We're about a tenth of the energy required to move the data as electricity," Saleh says. "If you're talking about one chip, that's not a big deal, but you start talking about someone like Google or Microsoft that has a million CPUs in a data center, and then they have tens of those data centers...." Doing the math, converting the world's data centers to optical switching could mean a savings of up to five percent of the world's energy.
"It's really about more data, lower power, lower cost. All the key metrics that you think of in data center operation get improved by photonics," he says. "This to me is the foundational technology that's going to be required to enable the next wave of innovation that comes through the internet and beyond. When I hear things like ‘metaverse,' AI and ML, for me, none of those things are possible without a complete transformation of the underlying infrastructure and how that infrastructure communicates."
He makes an analogy with the US interstate highway system, which moves all major commerce. "[Photonics] opens up those freeways, those interstates, all the way into the core chip. We think it's a wholesale transformation."
Photonic computing isn't a wholly limitless frontier, however: There are some inherent constraints. Because the calculations performed by photonic chips such as Envise are analog rather than digital, they can be somewhat less accurate than conventional transistors, and system noise can also be a problem. Photonic devices such as MZIs also tend to be larger and can't yet be packed on a chip as densely as more traditional electronic components.
Although designers are working diligently to overcome such obstacles, it's unlikely that all computers are going to go fully photonic anytime soon. "It's not going to be in your cell phone," says Harris. Optical computing, he predicts, will first be more of a high-performance AI accelerator for use by financial institutions, retail businesses, the military, and autonomous vehicle makers.
With AI systems striving for ever greater computer power, photonics computing technology offers a way around the inexorable technical limits of Moore's law. Nicholas Harris offers an inside joke at Lightmatter: "Moore's law? No problem."
Mark Wolverton is a freelance science writer and author based in Philadelphia.
Enjoy this article? Get similar news in your inbox |
|