Cluttered and complex
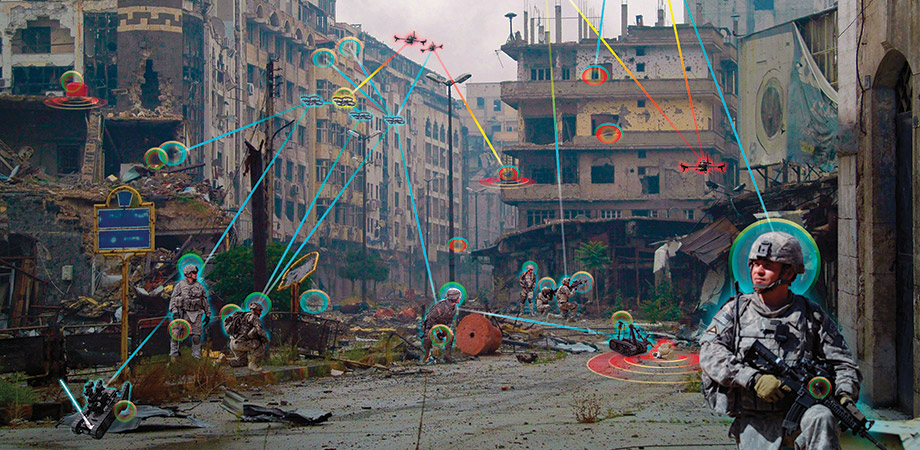
United States Department of Defense (DoD) senior leaders believe that artificial intelligence (AI) will play a critical role in the future success of multidomain operations (MDO). These can cut across cyber, land, air, space, and sea and bring together resources from the Air Force, Army, and Navy, as well as international coalition partners. Two primary challenges in this cluttered and complex battlespace are the need to collect, analyze, and disseminate information, coupled with the ability to make decisions.
Led by the commercial sector, the recent explosion of AI, primarily based on machine learning (ML), began in the early 2000s with data from successful deployment of wireless networking and connectivity, and the widespread availability of computational resources. In the last decade, this explosion was further propagated by the confluence of AI algorithms focused on data conditioning, modeling, classification, and regression; large data sets focused on collection, storage, and retrieval; and fast and cheap computing on CPUs, TPUs, and GPUs.
The commercial sector landscape for AI, which used to be niche, now spans a wide range of applications, industries, and a complete technology stack. Applications include enterprise intelligence, enterprise functions, autonomous systems, and agents such as robots and drones. Industries utilizing AI now include agriculture, education, finance and investment, legal, logistics, materials, retail, and healthcare. The technology stack includes agent enablers, data science, ML, natural language processing, data capture, storage, retrieval, open-source libraries, and computing hardware.
The Defense Department can, and does, leverage these decades of commercial investment and know-how learned from applications. However, military domains are also distinct from commercial applications and pose unique challenges. Rapidly changing battle space situations are characterized by limited access to real data to train AI, and peer adversaries who employ deceptive techniques to inject confusion and chaos. These elements challenge AI algorithms that rely on labeled datasets, crowdsourced dataset labeling, and metrics to benchmark the dataset.
Moreover, these MDO information and decision challenges require convergence of capabilities across multiple echelons at speeds and scales beyond human cognition. It is a driving force for the military to adopt AI-enabled technologies that will address these gaps with the goal of incorporation into their operations. Unlike commercial applications focused on channel-to-market, military applications must also align with defense doctrine, organization, training, supplies and equipment, leadership, education, personnel, and facilities.
To achieve this vision in MDO environments the military must be successful in addressing large-scale technical challenges. These include distributed operations in complex settings; extreme resource constraints such as weak communications or computational power; learning in complex data environments with sparse and potentially compromised data samples or highly contested environments; and relying on rapidly adaptable teams of autonomous AI systems that interact and learn from high-level mission goals set by people. At the tactical edge, this will require AI that is reliable and safe, explainable, robust to multiple varying adversarial attacks, and adaptive to evolving environments and mission tasks.
With the widespread interest in AI and the applications to data, a practitioner has a vast array of tools across the technology stack that enable AI systems built for civilian applications. These commercial platforms also offer democratization of AI with entire support ecosystems built around the platforms, which has contributed to the acceleration of AI adoption.
The introduction of AI tools as canned algorithms represents a subtle but important shift. In the past, the utilization of AI usually required carefully schematized data to fit a custom-designed application. Now, data representations and schemata are more likely to be taken off-the-shelf and pipelined into these canned algorithms. While this makes AI more easily and widely applicable, it also obscures assumptions made in the design of the data schema, which can lead to unseen biases and oversights. That is why the results from an AI system can be difficult to explain and necessitates continued exploration of systems operation on a theoretical level.
In most applications, AI practitioners comment that the actual work focuses on conditioning and preparing the data, or even constructing the databases themselves, with the actual application of AI algorithms as a limited part of making these automatic methods succeed.
What is more, AI systems must contend with system test and evaluation, validation, and verification. Challenges include lack of a well-defined performance space and lack of trust. Predictability and lack of transparency in AI systems (because learning models are often a black box) can create issues, too. For example, when AI-based systems, especially with their highly nonlinear behavior, collect and analyze data, they may inadvertently create information that is protected with no recourse for understanding information provenance.
Finally, for DoD applications, consequence of actions must be considered. AI systems used for warfare may conflict with the ethics of private companies that would otherwise develop these algorithms.
Despite many obstacles, AI tools are expected to be crucial to military operations, now and in the future, so these problems are worth solving.
Retired US General John E. Hyten, the recent vice chairman of the Joint Chiefs of Staff, agrees. He says, "If we go into a future where there are no lines on the battlefield, and we have ubiquitous all-domain command and control and logistics that go seamlessly from place to place, from service to service, and it all happens in enormous speed... I can't figure out in my mind how to do that without artificial intelligence."
The upcoming conference on Artificial Intelligence and Machine Learning for Multi-Domain Operations Applications at SPIE Defense + Commercial Sensing will dive deeply into the questions raised in this article. The story was written by Conference Chairs Tien Pham and Latasha Solomon (both from the Army Research Lab), Conference Co-Chair Ravi Ravichandran (BAE Systems), and Program Committee member Danda B. Rawat (Howard University).
Enjoy this article? Get similar news in your inbox |
|