Clinical translation of machine learning for medical imaging
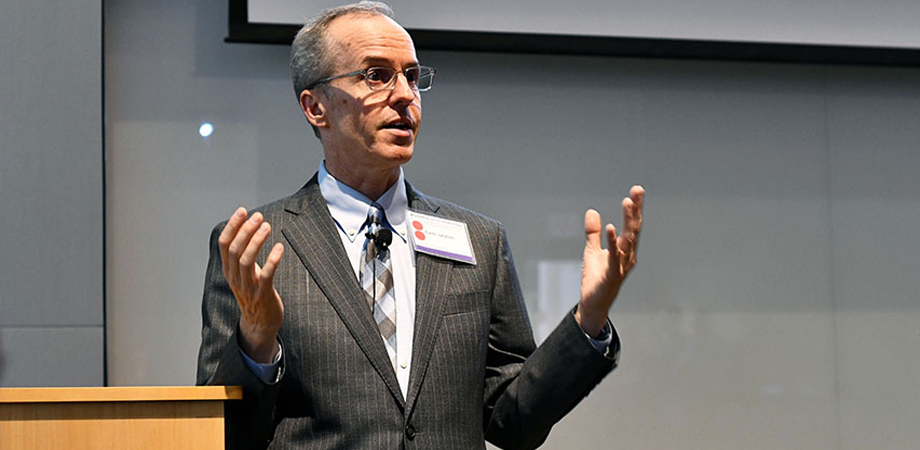
At Stanford University, Curtis Langlotz has a variety of roles that give him a view of artificial intelligence (AI) that ranges from the lab to the bedside. Langlotz is a professor of radiology, medicine, and biomedical data science at Stanford and director of the university’s Center for Artificial Intelligence in Medicine and Imaging (AIMI Center).
“My role as director of the AIMI Center gives me the privilege of supporting over 150 affiliated faculty from 20 departments at Stanford who are dedicated to the use of machine learning to improve patient care,” says Langlotz. “I also run an NIH-funded lab with about a dozen students and post-docs. It is so rewarding to be exposed to their incredible energy and see the results of their creative intellect.”
If that’s not enough, Langlotz also has a role with Stanford Health Care helping to set strategy for the information technology that runs Stanford’s radiology practice. And — he still reads chest X-rays two shifts per week.
What are some of the projects at your lab that you’re most excited about?
I am super excited about our work on foundation models. We have collaborated with Pranav Rajpurkar’s lab on the RadGraph entity and relation extraction algorithm and have paired that with GPT-4 out of the box to create a system that can explain concepts in radiology reports to patients at a reading level and in a language that they prefer.
Probably the most exciting work is collaborating with Akshay Chaudhari’s lab. We are working toward a foundation model trained on the entire Stanford PACS — over 1.5 petabytes of images and reports. The first step in that journey was recently published. If you look at the size of training datasets for foundation models inside and outside of medicine, there is a huge deficit in size so far in medicine. We have a long way to go. We haven’t yet had a “ChatGPT moment” in healthcare, where models trained on massive datasets show unexpected performance on a range of tasks, right out of the box.
What do you see as the most important aspect of your research at this time?
That’s a hard question, like “which of your children do you love the most?” I will answer it this way: The most important thing is that we have built an interdisciplinary team that works well together on these large, impactful projects.
What led to your interest in working with AI?
I took my first AI class as an undergraduate at Stanford in the early 1980s and immediately fell in love with it. I had been reading the Pulitzer Prize winning book, Gödel, Escher, Bach, at the time, and everything clicked. I pursued AI for my master’s and my PhD, back when our ideas of how it might affect the practice of medicine were very abstract and distant. I am gratified that the new machine learning approaches are finally having a significant impact on how we practice — and it’s only the beginning!
Some in the medical imaging community worry that AI will replace them. How do you address that issue with those who feel this way?
Peak fear among radiologists was about five years ago. Many computer science experts and other pundits at that time thought it would be easy to replace us. I wrote an editorial in 2019 giving all the reasons why it wasn’t going to happen. Most radiologists realize now, based on their experiences with AI, that our skills are hard to replicate, and that we do much more than just find abnormalities on images. I guess my top reason is that our data is different and more complex.
Outside medicine, computer vision started with ImageNet, which contained 224x224 images. Now we have diffusion systems like DALL-E that produce 1024x1024 images. But that’s the size of just a single CT slice! Each sequence has 100 of those, and we typically obtain multiple sequences and need to compare to the priors. And for MRI, we might have not just 3-4 sequences, but 20 or more. And we’re not looking for dogs or cats, but for extremely subtle abnormalities. Those complexities lead to so many interesting research questions!
What do you see as the future of AI in medical imaging?
I just wrote an editorial giving 10 predictions for the future of AI — I won’t repeat them all here. But the first prediction on the list is that radiology will continue to lead the way. Three quarters of FDA-cleared machine learning algorithms target radiology practice, probably because we have so much digital data and neural nets are really good at processing images. So AI in our specialty already constitutes a growing industry, with over 100 companies and hundreds of cleared algorithms.
The future of imaging AI products will be focused more on algorithms that save physicians time or solve operational problems. My current favorite-use case is algorithms that can automatically draft reports, much like residents do at many academic institutions — a huge help and major productivity advantage.
What would you like attendees to learn from your talk at SPIE Medical Imaging?
I presented some of my earliest radiology informatics research work at SPIE when I was still a resident. So, I am delighted to return and have a chance to see many old friends. Back then we were studying the financial and operational impact of PACS and asking whether they were cost effective to implement. It’s funny to reflect back on those questions — the answers are so obvious now. When I speak next week, I am looking forward to giving the audience a sense of the future of AI in medical imaging, the key AI research themes, the paths to clinical impact, the role of foundation models, and some of the pitfalls to watch for.
Enjoy this article? Get similar news in your inbox |
|